US-China Compete To Dominate Artificial Intelligence
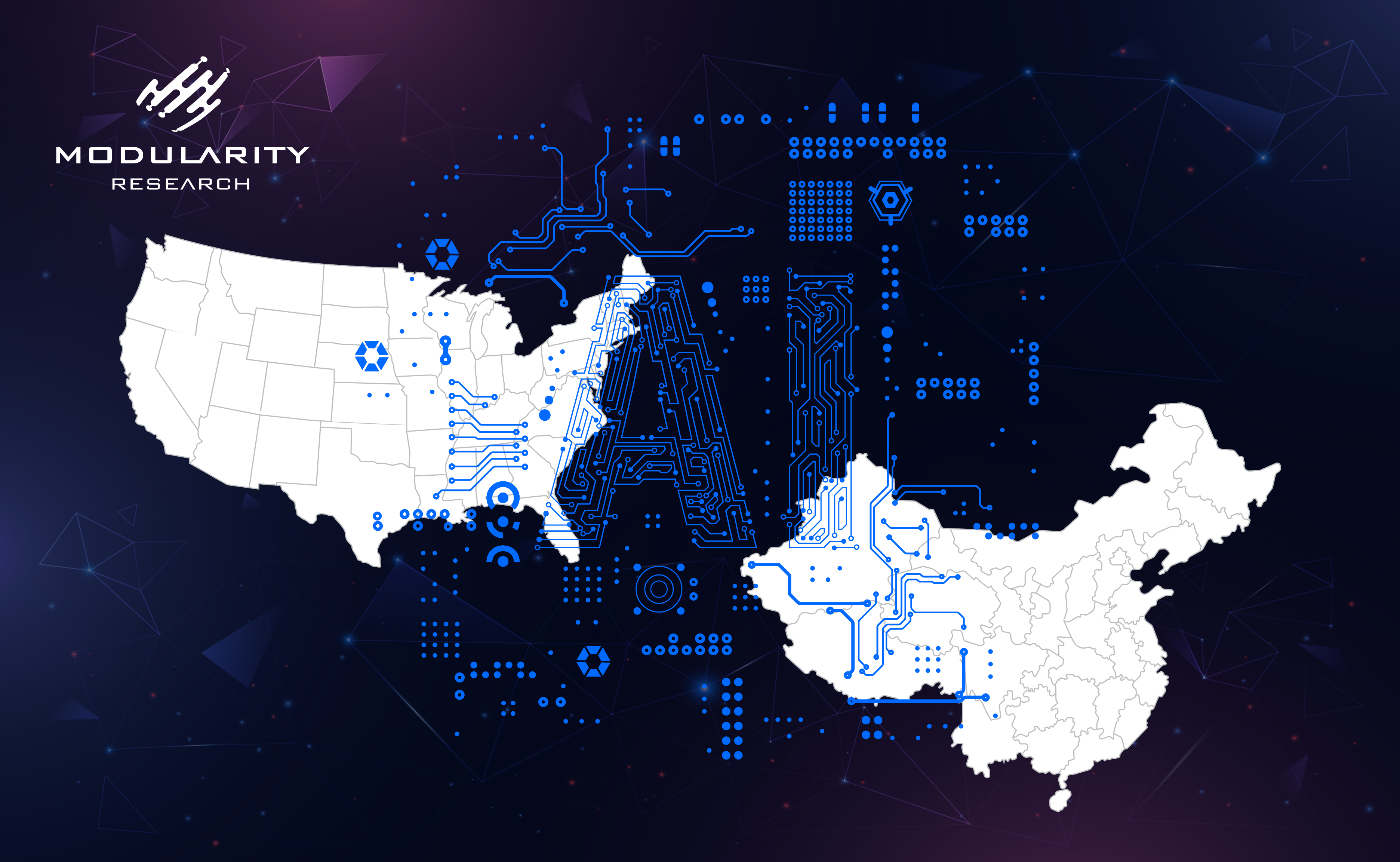
The race between the United States and China in artificial intelligence (AI) is multifaceted, involving a complex value chain that encompasses everything from semiconductor chips to commercial AI applications. Understanding this competition requires a detailed examination of each component of the AI value chain, the strengths and weaknesses of each country, and the policy implications shaping the trajectory of this global rivalry.
The AI Value Chain: From Chips to Applications
The AI ecosystem can be broken down into several key stages:
- Semiconductor Chips: The foundational hardware enabling AI systems.
- Cloud Computing Infrastructure: The platforms powering AI model training and deployment.
- Development Frameworks: Tools and software for creating AI models.
- AI Models: Pre-trained or application-specific machine learning systems.
- Commercial Applications: Consumer and enterprise solutions derived from AI models.
1. Semiconductor Chips: US Dominance
China remains highly dependent on US-designed chips for AI applications. Estimates suggest that 95% of the chips optimized for AI—such as Nvidia GPUs, AMD GPUs, and Google TPUs—are designed by US companies. This reliance places China at a significant disadvantage in upstream AI development, despite ongoing efforts to reduce this dependency.
2. Cloud Computing: A Competitive Playing Field
In cloud computing, Chinese companies like Tencent and Alibaba are considered peers of US giants such as Microsoft Azure and Amazon Web Services (AWS). While these Chinese firms may not always win market share, particularly in regions like India, they are recognized as formidable competitors capable of challenging US dominance.
3. Development Frameworks: A Critical Weakness for China
Machine learning development frameworks, such as PyTorch and TensorFlow, are predominantly US-led. Although open source, these frameworks are heavily influenced by US institutions and companies. China's lack of a strong domestic framework is a recognized gap, posing challenges for its AI ecosystem.
4. AI Models: Large Language Models vs. Application-Specific AI
Large Language Models and Foundation Models
Foundation models, including large language models, represent a transformative shift in AI. Unlike traditional AI, which required vast, application-specific datasets (e.g., millions of face images for facial recognition), foundation models can generalize capabilities. By training on massive datasets, these models can be fine-tuned for specific applications with minimal additional data.
While Chinese companies, such as Baidu with its Ernie Bot, are pursuing foundation models, they face significant hurdles. One key challenge is the relative scarcity of openly accessible Chinese-language text compared to English-language text, which is more readily available for training on the open internet. This discrepancy often forces Chinese firms to train on English data and rely on translation for localized applications.
Application-Specific AI
In more traditional AI domains, such as image recognition and voice recognition, Chinese companies are world-class competitors. They frequently excel in international benchmarks and have strong enterprise applications. However, expanding globally remains a challenge due to geopolitical and trust-related concerns.
5. Commercial Applications: Innovation vs. Global Expansion
China's innovation in AI applications is undeniable. From voice recognition to same-day delivery logistics, Chinese companies have demonstrated their ability to lead in complex technologies. However, Chinese firms often struggle to expand internationally, particularly in regions where trust in Chinese technology remains a barrier.
US and Private Sector Perspectives on China's AI Progress
Divergent Views in US Government
The US government lacks a unified stance on China's AI capabilities. Opinions range from alarmist fears of Chinese AI dominance to pragmatic recognition of China's technological prowess. National security concerns dominate the discourse, with policymakers grappling with how to balance commercial collaboration and military containment.
Private Sector Recognition
US companies and researchers widely acknowledge China as a peer competitor in AI. Chinese researchers are a prominent presence at major AI conferences, and Chinese firms frequently win international competitions. This recognition underscores China's position as a close second to the United States, far ahead of other competitors.
The Policy Tightrope: Enabling Commerce vs. Limiting Military Modernization
A key challenge for the US lies in enabling commercial AI collaboration without inadvertently advancing China's military capabilities. China's stated aim of integrating commercial and military advancements complicates this task. The October 7 export controls exemplify the US government's attempt to address this dilemma, marking a significant shift in US-China relations.
Conclusion
The US-China AI competition is a nuanced and evolving landscape. While the United States maintains clear advantages in upstream elements like semiconductor design and development frameworks, China is highly competitive in application-specific AI and certain commercial innovations. However, geopolitical barriers and structural challenges, such as limited access to open training data, constrain China's ability to fully match US capabilities.
The outcome of this rivalry will depend not only on technological advancements but also on the strategic policies and global collaborations each country pursues. As the race intensifies, understanding these dynamics will be critical for navigating the future of AI.